
🔬💊 Imagine a world where life-saving drugs are developed in a fraction of the time and at a fraction of the cost. This isn’t science fiction; it’s the revolutionary reality being shaped by Artificial Intelligence (AI) and Machine Learning (ML) in the field of drug discovery. The biopharmaceutical industry is on the cusp of a paradigm shift, and the catalyst is the integration of these cutting-edge technologies.
For decades, drug discovery has been a painstakingly slow and expensive process, often taking years and billions of dollars to bring a single medication to market. But what if we told you that AI and ML are completely revolutionizing this landscape? 🚀 These powerful tools are not just streamlining processes; they’re reimagining the entire approach to drug discovery and development.
In this blog post, we’ll dive deep into the transformative impact of AI and Machine Learning on drug discovery. From accelerating research timelines to enhancing target identification, from revolutionizing drug design to leveraging big data – we’ll explore how these technologies are reshaping the biopharmaceutical industry. We’ll also examine the challenges, collaborative ecosystems, and exciting future prospects in this rapidly evolving field. Get ready to discover how AI and ML are not just changing the game – they’re rewriting the rules of drug discovery. 💡🧬
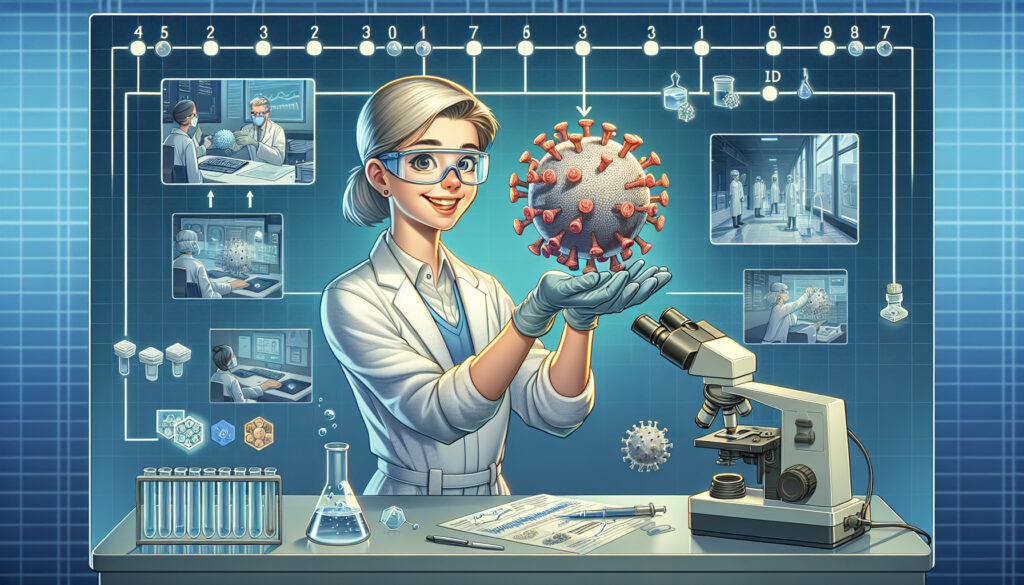
Understanding AI and Machine Learning in Drug Discovery
Defining AI and ML in the context of biopharmaceuticals
Artificial Intelligence (AI) and Machine Learning (ML) are revolutionizing the biopharmaceutical industry, particularly in drug discovery. AI refers to computer systems that can perform tasks that typically require human intelligence, while ML is a subset of AI that enables systems to learn and improve from experience without being explicitly programmed.
In the context of biopharmaceuticals, AI and ML are being used to analyze vast amounts of biological and chemical data, identify patterns, and make predictions that can significantly accelerate the drug discovery process. These technologies are transforming how researchers approach target identification, lead optimization, and clinical trial design.
Key technologies driving innovation
Several key technologies are driving innovation in AI-powered drug discovery:
- Deep Learning: A subset of ML that uses neural networks to analyze complex data structures
- Natural Language Processing (NLP): Enables machines to understand and interpret scientific literature
- Computer Vision: Allows for the analysis of medical images and molecular structures
- Reinforcement Learning: Helps in optimizing drug design through iterative processes
Here’s a comparison of these technologies and their applications in drug discovery:
Technology | Application in Drug Discovery |
---|---|
Deep Learning | Predicting drug-target interactions, molecular property prediction |
NLP | Mining scientific literature for new insights, analyzing patient records |
Computer Vision | Analyzing cellular images, predicting protein structures |
Reinforcement Learning | Optimizing molecular structures, designing novel compounds |
Advantages over traditional drug discovery methods
AI and ML offer several significant advantages over traditional drug discovery methods:
- Speed: AI can process and analyze vast amounts of data in a fraction of the time it would take human researchers.
- Cost-effectiveness: By reducing the time and resources required for early-stage drug discovery, AI can significantly lower overall development costs.
- Improved accuracy: ML algorithms can identify patterns and relationships that might be missed by human researchers, leading to more accurate predictions.
- Scalability: AI systems can easily scale to handle larger datasets and more complex problems as they arise.
- Novel insights: AI can uncover unexpected connections and generate hypotheses that human researchers might not have considered.
These advantages are particularly evident in the following areas:
- Target identification: AI can analyze genomic and proteomic data to identify novel drug targets more quickly and accurately.
- Lead optimization: ML algorithms can predict the properties of potential drug candidates, allowing for faster optimization of lead compounds.
- Repurposing existing drugs: AI can identify new therapeutic applications for existing drugs by analyzing their molecular properties and potential interactions.
As we delve deeper into the impact of AI and ML on drug discovery, we’ll explore how these technologies are accelerating the entire drug development process, from initial research to clinical trials.
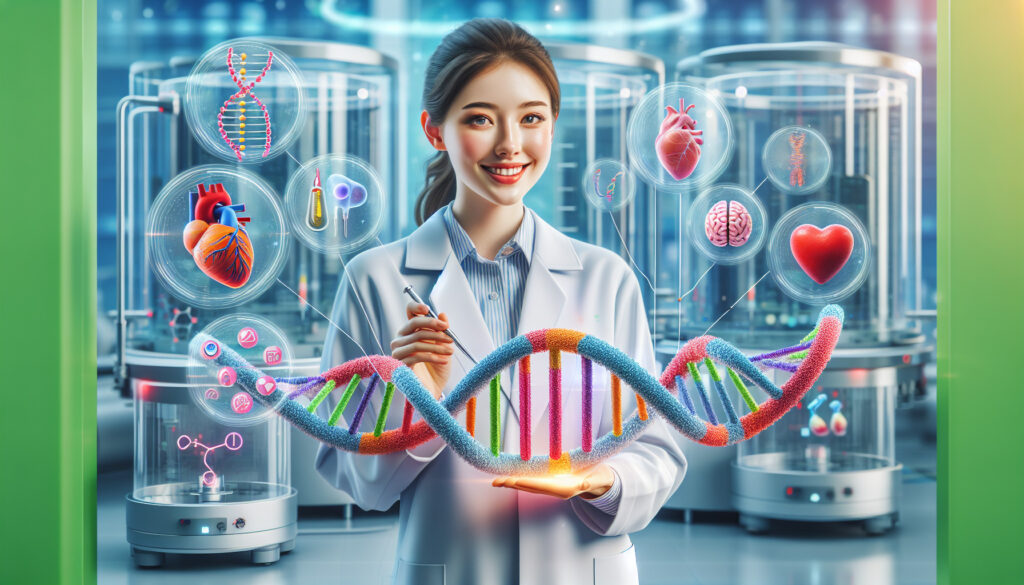
Accelerating the Drug Discovery Process
The integration of AI and machine learning in drug discovery has revolutionized the traditionally time-consuming and costly process. By leveraging these advanced technologies, biopharmaceutical companies are significantly accelerating various stages of drug discovery, from initial screening to final development.
Faster screening of potential drug candidates
AI-powered algorithms can rapidly analyze vast libraries of chemical compounds, identifying potential drug candidates in a fraction of the time it would take using conventional methods. This accelerated screening process allows researchers to:
- Evaluate millions of compounds in days rather than months
- Identify promising leads more efficiently
- Reduce the overall cost of initial screening
Traditional Screening | AI-Powered Screening |
---|---|
Months to years | Days to weeks |
Limited compound analysis | Millions of compounds analyzed |
High cost | Significantly reduced cost |
Labor-intensive | Automated and efficient |
Improved prediction of drug efficacy and safety
Machine learning models trained on extensive datasets can predict a compound’s efficacy and safety profile with remarkable accuracy. This capability enables:
- Early identification of potential side effects
- More accurate estimation of therapeutic potential
- Reduction in late-stage clinical trial failures
By improving predictions, AI helps researchers focus on the most promising candidates, reducing time and resources spent on less viable options.
Optimizing molecular structures for better outcomes
AI algorithms can suggest modifications to molecular structures to enhance their therapeutic properties. This optimization process involves:
- Analyzing structure-activity relationships
- Predicting how structural changes affect drug properties
- Generating novel molecular designs with improved characteristics
Through iterative optimization, researchers can develop more effective and safer drug candidates in less time than traditional methods.
Reducing time-to-market for new medications
The cumulative effect of AI-driven acceleration across various stages of drug discovery significantly reduces the overall time-to-market for new medications. This reduction is achieved through:
- Streamlined target identification and validation
- Faster lead optimization
- More efficient preclinical testing
- Improved clinical trial design and patient selection
As a result, potentially life-saving medications can reach patients faster, addressing unmet medical needs more rapidly than ever before.
The impact of AI and machine learning on accelerating drug discovery is profound, offering hope for faster development of treatments for complex diseases. With these advancements, the pharmaceutical industry is poised to make significant strides in improving global health outcomes. As we explore further, we’ll see how AI is enhancing the crucial process of target identification and validation in drug discovery.

Enhancing Target Identification and Validation
Now that we’ve explored how AI accelerates the drug discovery process, let’s delve into its role in enhancing target identification and validation. This crucial step in drug development has been significantly improved by the integration of AI and machine learning technologies.
AI-powered analysis of biological data
AI algorithms excel at analyzing vast amounts of biological data, including genomic, proteomic, and metabolomic information. This capability allows researchers to identify potential drug targets with unprecedented speed and accuracy. Machine learning models can sift through complex datasets to recognize patterns and correlations that might be overlooked by human researchers.
Here’s a comparison of traditional vs. AI-powered biological data analysis:
Aspect | Traditional Analysis | AI-Powered Analysis |
---|---|---|
Speed | Slow, manual process | Rapid, automated analysis |
Data volume | Limited by human capacity | Can handle massive datasets |
Pattern recognition | May miss subtle correlations | Identifies complex patterns |
Bias | Subject to human bias | Reduces bias through objective analysis |
Cost | Time-consuming and expensive | More cost-effective in the long run |
Uncovering novel drug targets
One of the most exciting applications of AI in target identification is its ability to uncover novel drug targets. By analyzing diverse datasets, including scientific literature, clinical trial data, and patient records, AI can identify previously unknown connections between genes, proteins, and diseases.
AI algorithms can:
- Predict protein structures and functions
- Identify potential binding sites
- Analyze gene expression data to find new therapeutic targets
- Suggest repurposing opportunities for existing drugs
This approach has led to the discovery of several promising drug targets that were not apparent through traditional research methods.
Predicting protein-ligand interactions
Once potential targets are identified, AI plays a crucial role in predicting protein-ligand interactions. This step is vital for understanding how a drug molecule might bind to its target and exert its therapeutic effect.
Machine learning models, trained on extensive databases of known protein-ligand interactions, can:
- Predict binding affinities
- Simulate molecular docking
- Identify potential off-target effects
- Optimize lead compounds for better target engagement
These predictions help researchers prioritize the most promising drug candidates, reducing the time and resources spent on less viable options.
By leveraging AI in target identification and validation, pharmaceutical companies can significantly enhance the efficiency and success rate of their drug discovery efforts. This approach not only accelerates the process but also increases the likelihood of finding innovative treatments for complex diseases.
Next, we’ll explore how AI is revolutionizing drug design and development, building upon these enhanced target identification techniques.

Revolutionizing Drug Design and Development
As we delve deeper into the impact of AI and machine learning on drug discovery, we now turn our attention to how these technologies are revolutionizing drug design and development. This transformation is reshaping the entire process, from initial concept to clinical trials.
De novo drug design using AI algorithms
AI algorithms are enabling de novo drug design, a process where entirely new molecules are created from scratch. This approach leverages machine learning models trained on vast databases of known compounds and their properties. By understanding the relationships between molecular structures and their biological activities, AI can generate novel molecules with desired characteristics.
- Benefits of AI in de novo drug design:
- Rapid exploration of chemical space
- Design of molecules with specific target properties
- Reduction in time and cost of initial drug discovery phases
Repurposing existing drugs for new applications
AI is also revolutionizing drug repurposing, identifying new therapeutic uses for existing drugs. This approach is particularly valuable as it can significantly reduce the time and cost associated with bringing a new treatment to market.
Traditional Drug Development | AI-Driven Drug Repurposing |
---|---|
10-15 years | 3-5 years |
$1-2 billion cost | $300-500 million cost |
High failure rate | Lower risk |
Machine learning algorithms can analyze vast amounts of data, including drug-target interactions, gene expression profiles, and clinical data, to identify potential new indications for existing drugs.
Personalized medicine approaches
AI is paving the way for more personalized medicine approaches in drug development. By analyzing individual patient data, including genetic information, lifestyle factors, and treatment histories, AI can help predict which drugs are most likely to be effective for specific patients.
- Key aspects of AI in personalized medicine:
- Identification of biomarkers for drug response
- Prediction of adverse drug reactions
- Optimization of drug dosing regimens
This personalized approach not only improves treatment outcomes but also reduces the likelihood of adverse effects, ultimately leading to more efficient and cost-effective healthcare.
Improving success rates in clinical trials
AI is significantly enhancing the efficiency and success rates of clinical trials. By analyzing historical trial data, patient records, and scientific literature, AI can help:
- Identify optimal patient populations for specific trials
- Predict potential side effects and safety issues
- Design more efficient trial protocols
- Monitor and analyze trial data in real-time
These AI-driven improvements are leading to faster, more cost-effective clinical trials with higher success rates. This is crucial in bringing new treatments to patients more quickly and efficiently.
As we move forward, the integration of AI and machine learning in drug design and development continues to accelerate. Next, we’ll explore how big data is fueling these AI-driven innovations in biopharmaceuticals.

Big Data and AI in Biopharmaceuticals
The intersection of big data and artificial intelligence is revolutionizing the biopharmaceutical industry, offering unprecedented opportunities for innovation and efficiency in drug discovery and development.
Leveraging vast biological datasets
AI and machine learning algorithms excel at processing and analyzing massive amounts of biological data, unlocking insights that were previously hidden or too complex for human researchers to discern. These vast datasets include:
- Genomic sequences
- Protein structures
- Metabolomic profiles
- Electronic health records
- Scientific literature
By leveraging these datasets, AI can identify patterns, predict outcomes, and generate hypotheses at a scale and speed unattainable by traditional methods. This capability is particularly valuable in:
- Identifying novel drug targets
- Predicting drug-target interactions
- Optimizing lead compounds
- Forecasting potential side effects
Integration of multi-omics data
One of the most powerful applications of AI in biopharmaceuticals is the integration of multi-omics data. This approach combines information from various “omics” fields, including:
- Genomics
- Proteomics
- Transcriptomics
- Metabolomics
- Epigenomics
By integrating these diverse datasets, AI can provide a more comprehensive understanding of biological systems and disease mechanisms. This holistic view enables researchers to:
- Identify complex biomarkers
- Uncover novel drug targets
- Develop personalized treatment strategies
Here’s a comparison of single-omics vs. multi-omics approaches:
Aspect | Single-omics | Multi-omics |
---|---|---|
Data sources | Limited to one “omics” field | Integrates multiple “omics” datasets |
Complexity | Lower | Higher |
Insights | Focused on specific biological aspects | Comprehensive view of biological systems |
Predictive power | Moderate | High |
Personalization potential | Limited | Extensive |
Real-time analysis of clinical trial data
AI is transforming the way clinical trials are conducted and analyzed. By processing real-time data from ongoing trials, AI algorithms can:
- Identify potential safety concerns earlier
- Optimize patient recruitment and retention strategies
- Predict trial outcomes with greater accuracy
- Suggest adaptive trial designs based on interim results
This real-time analysis capability allows pharmaceutical companies to:
- Make informed decisions faster
- Reduce the cost and duration of clinical trials
- Improve patient safety and experience
- Increase the likelihood of successful drug development
As we harness the power of big data and AI in biopharmaceuticals, we’re witnessing a paradigm shift in drug discovery and development. This transformation is not without challenges, however. In the next section, we’ll explore some of the obstacles facing AI-driven drug discovery and how the industry is working to overcome them.
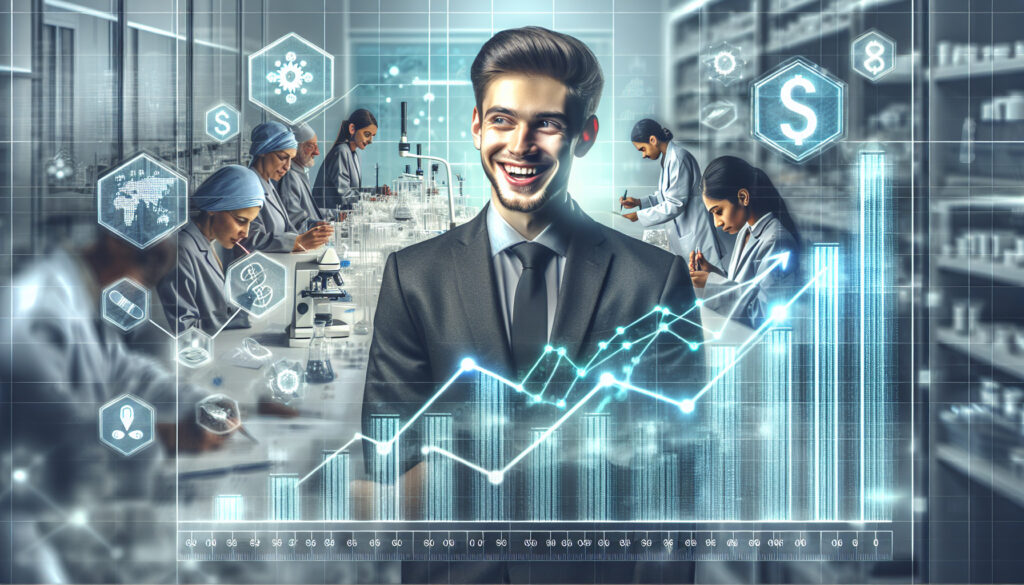
Overcoming Challenges in AI-Driven Drug Discovery
As AI and machine learning continue to revolutionize drug discovery, the biopharmaceutical industry faces several challenges that need to be addressed to fully harness the potential of these technologies. Let’s explore the key obstacles and potential solutions in AI-driven drug discovery.
Addressing data quality and bias issues
The quality and diversity of data used to train AI models are crucial for their effectiveness in drug discovery. However, several challenges arise:
- Data quality: Inconsistent or incomplete data can lead to unreliable predictions.
- Data bias: Historical data may contain biases that can perpetuate inequalities in drug development.
To overcome these challenges, researchers and companies are implementing:
- Rigorous data curation processes
- Diverse data collection strategies
- Advanced data cleaning techniques
- Collaborative data sharing initiatives
Challenge | Solution |
---|---|
Data quality | Implement strict quality control measures |
Data bias | Diversify data sources and use bias detection algorithms |
Limited data availability | Establish data sharing partnerships and use synthetic data generation |
Ensuring interpretability of AI models
While AI models can process vast amounts of data and identify patterns, their decision-making processes are often opaque. This “black box” nature can be problematic in drug discovery, where understanding the reasoning behind predictions is crucial. To address this:
- Develop explainable AI (XAI) techniques
- Use model-agnostic interpretation methods
- Implement visualization tools for AI decision pathways
Navigating regulatory frameworks
The rapidly evolving field of AI in drug discovery presents challenges for regulatory bodies. To ensure compliance and safety:
- Engage with regulatory agencies early in the development process
- Develop standards for AI model validation in drug discovery
- Create guidelines for AI-assisted clinical trial design
- Establish frameworks for continuous monitoring of AI-driven drug discovery processes
Ethical considerations in AI-driven research
As AI becomes more prevalent in drug discovery, ethical concerns arise:
- Privacy and data protection
- Equitable access to AI-driven healthcare innovations
- Potential job displacement in traditional research roles
To address these ethical challenges:
- Implement robust data anonymization techniques
- Develop ethical guidelines for AI use in drug discovery
- Invest in reskilling programs for researchers
- Ensure transparency in AI-driven decision-making processes
By addressing these challenges, the biopharmaceutical industry can unlock the full potential of AI and machine learning in drug discovery. This will lead to more efficient, cost-effective, and innovative approaches to developing life-saving medications. As we move forward, it’s crucial to consider how these advancements will shape the future of drug discovery and the broader healthcare landscape.
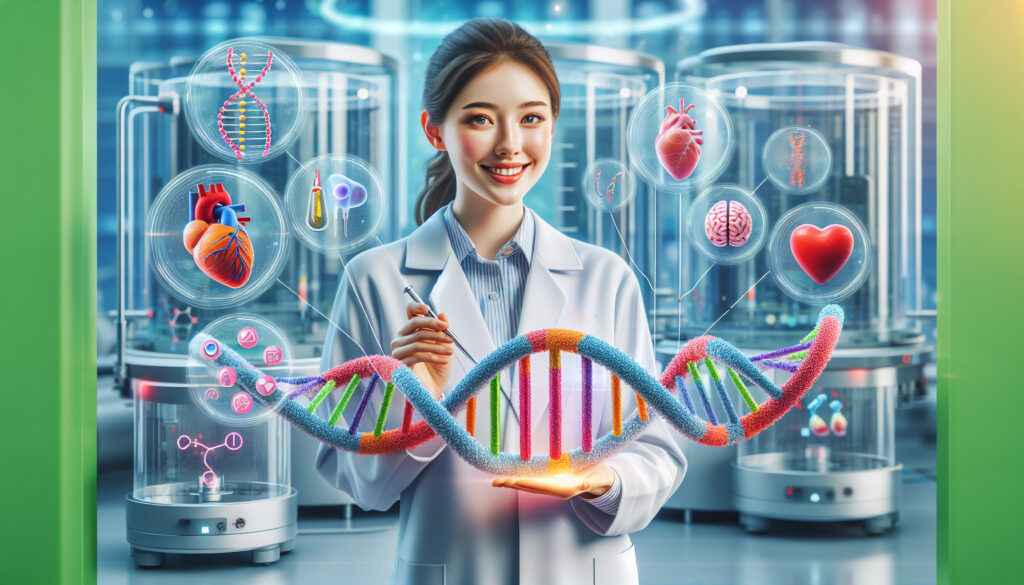
Collaborative Ecosystems in AI-Powered Drug Discovery
As we delve deeper into the world of AI-powered drug discovery, it’s clear that collaboration is key to unlocking its full potential. The complex nature of drug discovery requires diverse expertise and resources, making partnerships and collaborative ecosystems essential for success.
Partnerships between pharma and tech companies
The convergence of pharmaceutical expertise and technological innovation has given rise to powerful alliances between pharma and tech companies. These partnerships leverage the strengths of both sectors to accelerate drug discovery and development.
- Complementary skills: Pharma companies bring deep biological and medical knowledge, while tech firms offer cutting-edge AI and machine learning capabilities.
- Resource optimization: Shared resources lead to more efficient use of data, computational power, and research facilities.
- Faster innovation: Collaborative efforts often result in quicker breakthroughs and reduced time-to-market for new drugs.
Pharma Company | Tech Partner | Focus Area |
---|---|---|
GSK | DeepMind | Protein folding prediction |
AstraZeneca | BenevolentAI | Target identification |
Pfizer | IBM Watson | Drug repurposing |
Open-source platforms and data sharing initiatives
The spirit of collaboration extends beyond corporate partnerships, with open-source platforms and data sharing initiatives playing a crucial role in advancing AI-powered drug discovery.
- Open-source AI tools: Platforms like DeepChem and OpenAI Gym for Molecule Generation provide researchers with accessible tools for computational drug discovery.
- Data consortiums: Initiatives such as the COVID-19 Open Research Dataset (CORD-19) demonstrate the power of shared data in accelerating research.
- Blockchain for data sharing: Emerging blockchain technologies are enabling secure and transparent data sharing among multiple stakeholders.
These collaborative efforts not only democratize access to AI tools and data but also foster a global community of researchers working towards common goals in drug discovery.
Academic-industry collaborations
The synergy between academia and industry is another vital component of the collaborative ecosystem in AI-powered drug discovery.
- Knowledge transfer: Academic institutions provide cutting-edge research and novel algorithms, while industry partners offer real-world applications and datasets.
- Talent pipeline: Collaborations create opportunities for students and researchers to gain industry experience and contribute to practical drug discovery projects.
- Funding opportunities: Industry partnerships often lead to increased funding for academic research in AI and drug discovery.
Examples of successful academic-industry collaborations include the MIT-IBM Watson AI Lab and the Stanford-Google partnership for AI in genomics and drug discovery.
As we move forward, these collaborative ecosystems will continue to play a pivotal role in shaping the future of AI-powered drug discovery. By combining diverse expertise, resources, and data, the biopharmaceutical industry is poised to make unprecedented advances in developing life-saving therapies. Next, we’ll explore the exciting future prospects and emerging trends in this rapidly evolving field.
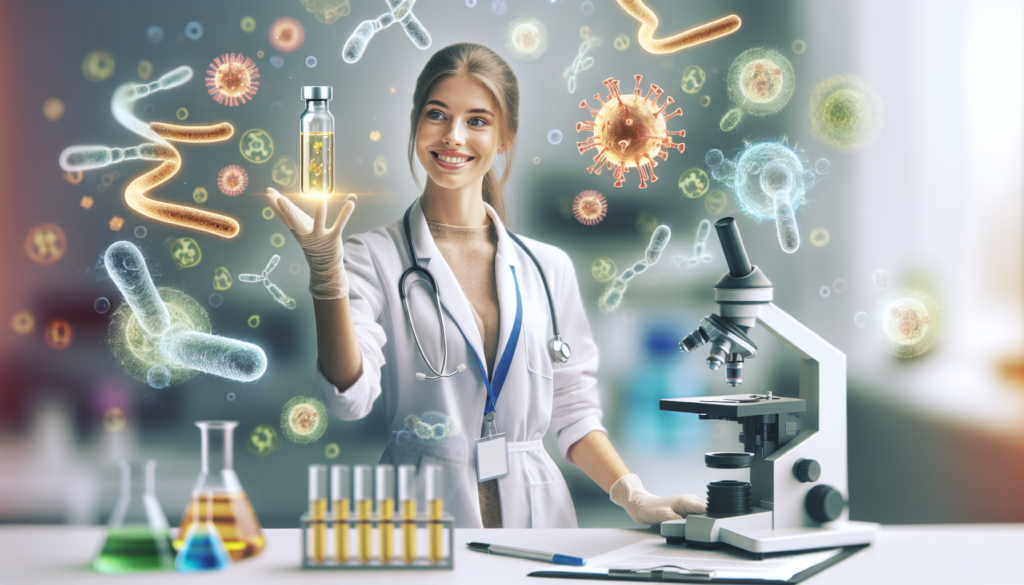
Future Prospects and Emerging Trends
As we look towards the horizon of AI and machine learning in drug discovery, several exciting developments are poised to revolutionize the biopharmaceutical industry. These advancements promise to further accelerate drug discovery, improve efficacy, and potentially unlock treatments for previously intractable diseases.
Quantum Computing in Drug Discovery
Quantum computing represents a paradigm shift in computational power, with profound implications for drug discovery. Unlike classical computers, quantum computers can process vast amounts of data simultaneously, making them ideal for complex molecular simulations and protein folding predictions.
- Advantages of quantum computing in drug discovery:
- Faster molecular simulations
- More accurate protein-ligand interactions
- Enhanced virtual screening capabilities
- Improved optimization of lead compounds
The integration of quantum computing with AI algorithms could lead to unprecedented accuracy in predicting drug-target interactions and identifying novel therapeutic candidates.
AI-Assisted Clinical Trial Design and Recruitment
AI is set to transform clinical trials, making them more efficient, cost-effective, and patient-centric. Machine learning algorithms can analyze vast amounts of historical trial data to optimize study designs and predict potential outcomes.
AI Application | Benefits in Clinical Trials |
---|---|
Patient Selection | More accurate identification of suitable participants |
Protocol Design | Optimization of trial parameters for better outcomes |
Real-time Monitoring | Early detection of safety issues or efficacy signals |
Data Analysis | Faster and more comprehensive analysis of trial results |
Moreover, AI-powered platforms can streamline patient recruitment by matching eligible participants with appropriate trials, potentially reducing the time and cost associated with this critical phase.
Integration of AI with Lab Automation
The convergence of AI and laboratory automation is creating “smart labs” that can operate with minimal human intervention. This integration promises to accelerate experimentation and data generation, crucial for drug discovery.
Key features of AI-integrated lab automation:
- Autonomous experiment design and execution
- Real-time data analysis and decision-making
- Predictive maintenance of lab equipment
- Optimization of resource allocation and workflow
This synergy between AI and automation not only increases throughput but also enhances reproducibility and reduces human error in experimental processes.
Potential for Breakthrough Discoveries in Complex Diseases
AI and machine learning hold immense promise for tackling complex diseases that have long eluded traditional drug discovery approaches. By analyzing multi-omics data and integrating diverse datasets, AI can uncover hidden patterns and novel therapeutic targets.
Areas where AI could drive breakthroughs:
- Neurodegenerative disorders (e.g., Alzheimer’s, Parkinson’s)
- Cancer heterogeneity and personalized treatments
- Rare genetic disorders
- Autoimmune diseases
AI’s ability to process and interpret vast amounts of biological data could lead to a deeper understanding of disease mechanisms and the identification of innovative treatment strategies.
As we move forward, the synergy between AI, quantum computing, and laboratory automation is set to redefine the landscape of drug discovery. These emerging trends not only promise to accelerate the development of new therapies but also offer hope for patients with previously untreatable conditions. The future of biopharmaceuticals is undoubtedly intertwined with the continued advancement of AI and machine learning technologies.
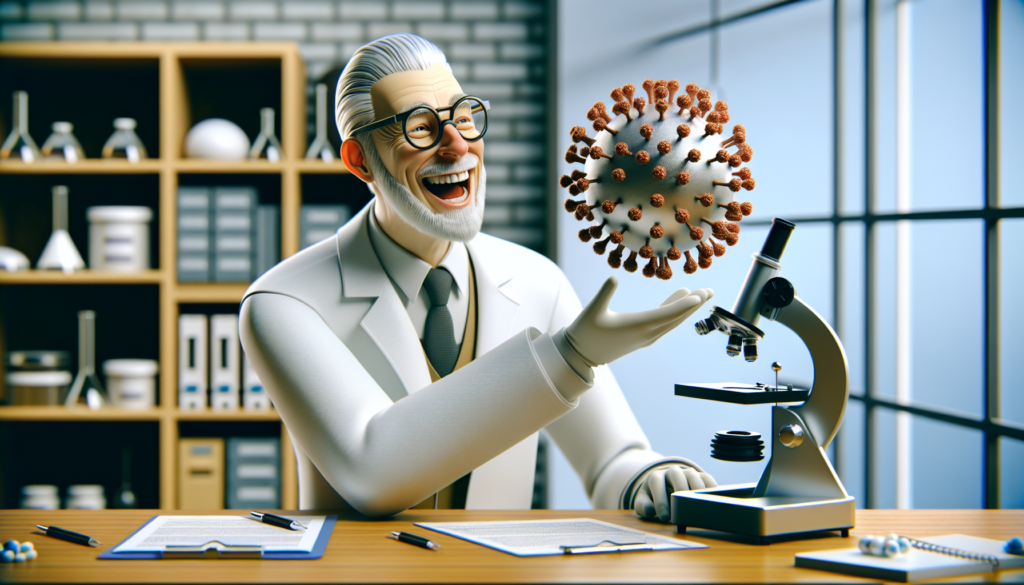
The integration of AI and machine learning in drug discovery has ushered in a new era for biopharmaceuticals. From accelerating the drug discovery process to enhancing target identification and revolutionizing drug design, these technologies are transforming every aspect of the industry. By harnessing the power of big data and overcoming challenges through collaborative ecosystems, AI-driven drug discovery is paving the way for more efficient, cost-effective, and innovative approaches to developing life-saving medications.
As we look to the future, the potential of AI and machine learning in biopharmaceuticals seems boundless. With emerging trends and ongoing advancements, we can expect even more groundbreaking discoveries and improved patient outcomes. Embracing these technologies and fostering collaborative partnerships will be crucial for pharmaceutical companies and researchers aiming to stay at the forefront of this revolution in drug discovery.