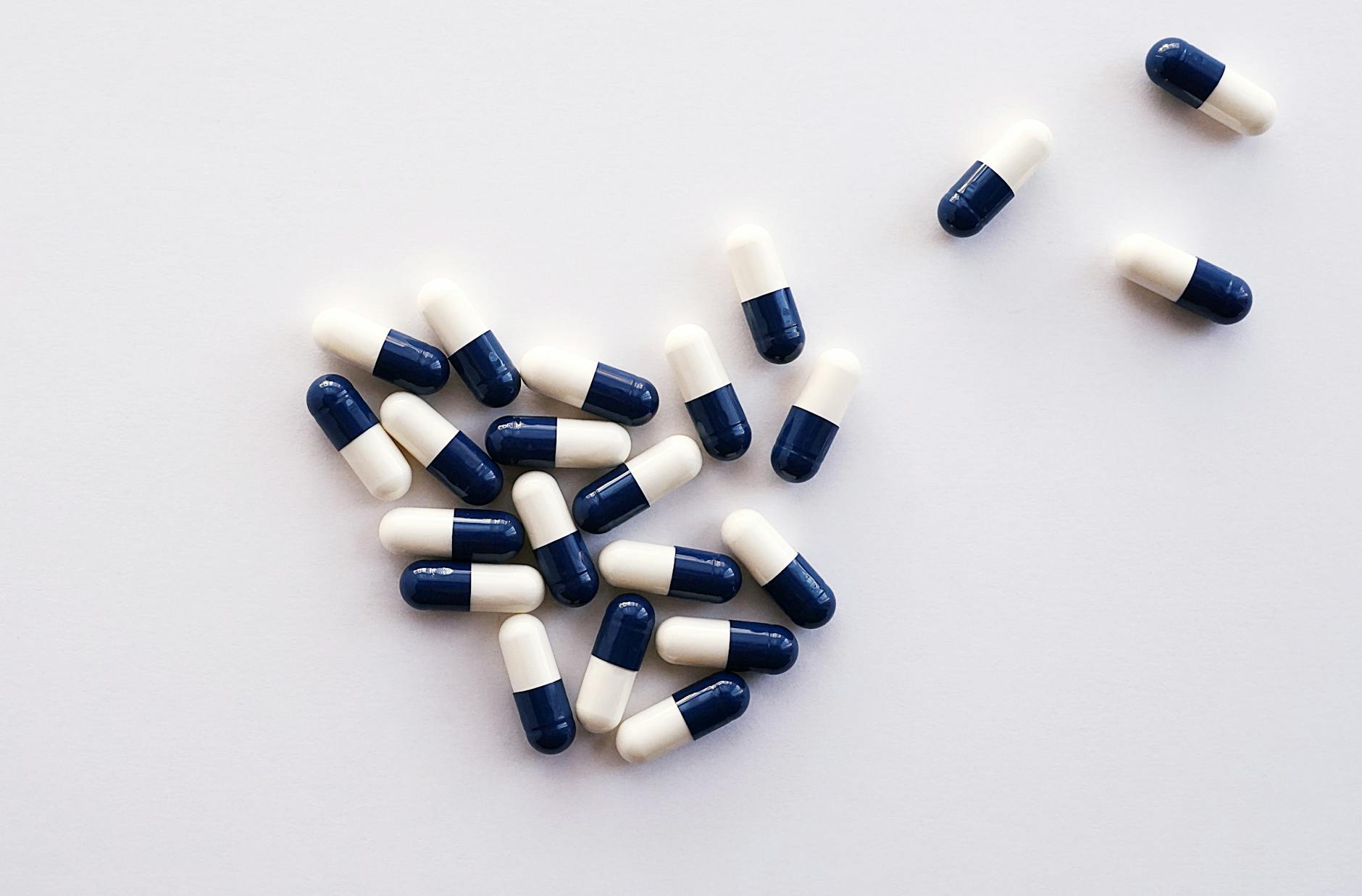
Artificial Intelligence (AI) is revolutionizing drug development, promising faster discoveries, more efficient processes, and groundbreaking treatments. 🧬💊 But as we race towards this exciting future, are we overlooking the ethical landmines that lie ahead? From privacy concerns to potential biases in AI algorithms, the integration of AI in pharmaceuticals raises serious questions about the balance between innovation and responsibility.
Imagine a world where life-saving drugs are developed in record time, but at what cost? 🤔 As AI delves deeper into our genetic data and medical records, the line between progress and privacy invasion blurs. The potential for AI to exacerbate social inequalities and dehumanize patient care looms large. Yet, the allure of AI’s capabilities in drug discovery is undeniable, with successful applications already emerging in cancer treatment, Alzheimer’s research, and antibiotic development.
In this blog post, we’ll explore the jaw-dropping ethics of AI in drug development, uncovering the risks that accompany its immense potential. We’ll dive into the ethical challenges, regulatory considerations, and strategies for responsible implementation. Join us as we navigate the complex landscape where cutting-edge technology meets age-old ethical dilemmas, and discover how we can harness AI’s power while safeguarding our values and humanity. 🚀🔬
The Promise of AI in Drug Development
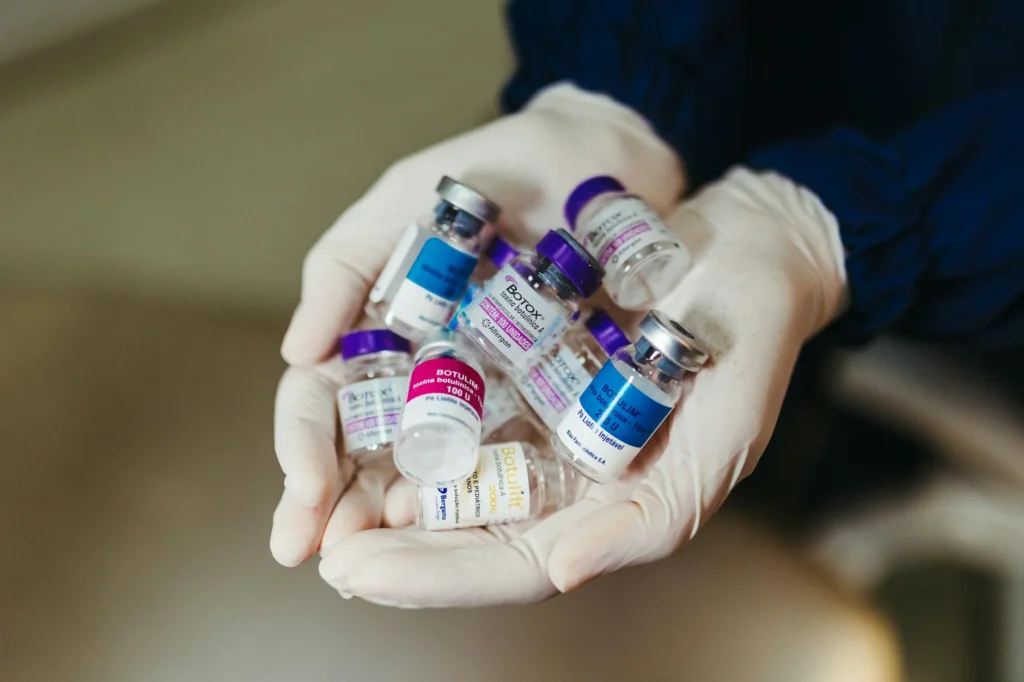
A. Enhancing efficiency and accuracy in drug discovery
Artificial Intelligence (AI) is revolutionizing the pharmaceutical industry by significantly enhancing the efficiency and accuracy of drug discovery processes. By leveraging machine learning (ML) and deep learning (DL) algorithms, AI can analyze vast datasets, identify patterns, and make predictions at an unprecedented speed and scale.
Key benefits of AI in enhancing drug discovery efficiency:
- Rapid analysis of large-scale data
- Improved target identification
- Accelerated screening of compounds
- Enhanced prediction of drug efficacy and toxicity
- Streamlined clinical trial processes
AI technologies enable researchers to process and interpret complex biological data more quickly and accurately than traditional methods. This capability allows for faster identification of potential drug targets and more efficient screening of candidate compounds.
B. Accelerating identification of novel compounds
One of the most promising applications of AI in drug development is its ability to accelerate the identification of novel compounds. By utilizing advanced algorithms and predictive modeling, AI can significantly reduce the time and resources required to discover potential drug candidates.
AI-Driven Approach | Traditional Approach |
---|---|
Rapid screening of millions of compounds | Manual screening of limited compounds |
Prediction of drug-target interactions | Experimental testing of interactions |
Automated analysis of structure-activity relationships | Manual analysis of structure-activity relationships |
Real-time optimization of lead compounds | Iterative optimization through multiple experiments |
AI has demonstrated remarkable success in identifying novel compounds for various diseases, including:
- Cancer treatment
- Alzheimer’s disease
- Antibiotic discovery
These case studies highlight AI’s potential to accelerate drug discovery timelines and expand the range of potential therapeutic agents.
C. Improving prediction of drug efficacy and toxicity
AI’s capability to process and analyze large datasets extends to improving the prediction of drug efficacy and toxicity. This advancement is crucial for reducing the failure rates in clinical trials and ensuring safer, more effective drugs reach the market.
AI-driven improvements in drug efficacy and toxicity prediction:
- Enhanced analysis of molecular structures and properties
- Improved understanding of drug-target interactions
- More accurate prediction of potential side effects
- Better assessment of drug metabolism and pharmacokinetics
- Increased success rates in clinical trials
By integrating AI with traditional experimental methods, researchers can optimize drug discovery outcomes and reduce the likelihood of late-stage failures in drug development.
AI also plays a significant role in personalized medicine, enabling the design of tailored therapies based on individual patient profiles. This approach has the potential to improve treatment outcomes and reduce adverse effects by matching patients with the most suitable drugs.
With these advancements in efficiency, accuracy, and predictive capabilities, AI is poised to transform the landscape of drug development. However, as we explore the promising applications of AI in pharmaceutical research, it’s crucial to consider the ethical implications and potential risks associated with this technology. In the next section, “Ethical Challenges in AI-Driven Drug Development,” we’ll delve into the complex considerations surrounding the use of AI in healthcare and drug discovery.
Ethical Challenges in AI-Driven Drug Development
Now that we have explored the promising potential of AI in drug development, it’s crucial to examine the ethical challenges that arise from this technological advancement. While AI offers tremendous benefits, it also presents significant concerns that must be addressed to ensure responsible and equitable implementation in healthcare.
Privacy concerns and data protection
The integration of AI in drug development raises substantial privacy concerns, particularly regarding patient data protection. As AI systems rely heavily on vast amounts of personal health information, safeguarding this sensitive data becomes paramount. According to a cross-sectional survey of pharmacy professionals in the Middle East and North Africa (MENA) region, 58.9% of respondents expressed apprehensions about patient data privacy.
Current legal frameworks, such as the General Data Protection Regulation (GDPR) in the EU and the Genetic Information Non-discrimination Act (GINA) in the US, aim to protect personal health data. However, these regulations may prove inadequate in the face of rapidly evolving AI technologies. The potential for data breaches and unauthorized access to patient information remains a significant challenge that requires ongoing attention and robust security measures.
Informed consent in AI-powered healthcare
Informed consent is a cornerstone of patient autonomy in healthcare, and its importance is magnified in the context of AI-driven drug development. Ensuring that patients fully understand and agree to the use of their data in AI systems is crucial. This challenge becomes even more complex when considering the following factors:
- The complexity of AI algorithms
- The potential long-term implications of data usage
- The evolving nature of AI applications in healthcare
To address these challenges, healthcare providers and researchers must develop clear, comprehensive, and accessible consent processes that empower patients to make informed decisions about their participation in AI-powered drug development initiatives.
Potential for bias and social inequality
AI systems in drug development are not immune to biases, which can lead to social inequalities and unfair treatment. Several key issues contribute to this ethical challenge:
- Underrepresentation of diverse populations in genomic databases
- Algorithmic biases that may perpetuate existing healthcare disparities
- Unequal access to AI-powered healthcare technologies
Bias Type | Description | Potential Impact |
---|---|---|
Data Bias | Underrepresentation of certain populations in training data | Reduced efficacy of drugs for underrepresented groups |
Algorithmic Bias | Inherent biases in AI models | Perpetuation of existing healthcare disparities |
Access Bias | Unequal distribution of AI-powered healthcare technologies | Widening gap in healthcare quality between socioeconomic classes |
To mitigate these biases, it is essential to prioritize diversity and inclusivity in AI-driven drug development processes. This includes ensuring representative data sets, implementing rigorous bias detection and correction mechanisms, and promoting equitable access to AI-powered healthcare solutions.
Furthermore, the potential impact of AI on healthcare professionals and job displacement must be considered. The survey of pharmacy professionals revealed that 62.9% of respondents were concerned about potential job displacement due to AI adoption. This underscores the need for careful planning and support for healthcare workers as AI technologies continue to advance.
As we move forward in exploring the ethical landscape of AI in drug development, it becomes clear that balancing the benefits and risks of this powerful technology is crucial. In the next section, we will delve into strategies for striking this delicate balance, ensuring that the advantages of AI are maximized while mitigating potential harms and preserving the essential human elements of healthcare.
Balancing AI Benefits and Risks
Now that we’ve explored the ethical challenges in AI-driven drug development, it’s crucial to examine how we can balance the benefits and risks associated with this revolutionary technology. The FDA’s comprehensive seven-step, risk-based framework provides valuable insights into achieving this balance.
A. Need for high-quality, diverse datasets
The foundation of effective AI in drug development lies in the quality and diversity of datasets used. The FDA emphasizes the importance of a robust data strategy in their framework:
- Develop a comprehensive data strategy
- Address potential biases in datasets
- Maintain data independence for validation
High-quality, diverse datasets are essential for:
- Reducing bias in AI models
- Enhancing the accuracy of predictions
- Ensuring broader applicability across different patient populations
B. Importance of human oversight and intervention
While AI offers powerful capabilities, human oversight remains critical. The FDA’s framework highlights the need for:
- Clear definition of the AI model’s Context of Use (COU)
- Assessment of model risk and potential consequences of errors
- Development and execution of a Credibility Assessment Plan
Human intervention is vital in:
Stage | Human Role |
---|---|
Planning | Defining questions and aligning with regulatory objectives |
Risk Assessment | Evaluating model influence on decision-making |
Execution | Monitoring and interpreting AI outputs |
Validation | Assessing model adequacy for intended use |
C. Integration of AI with traditional experimental methods
Balancing AI benefits and risks requires seamless integration with established drug development practices. The FDA’s guidance suggests:
- Combining AI model outputs with traditional experimental data
- Continuous monitoring of AI models, especially during manufacturing
- Establishing strong company-wide AI governance systems
This integration ensures:
- Comprehensive risk assessment
- Enhanced reliability of results
- Compliance with regulatory expectations
By adhering to these principles, pharmaceutical companies can leverage the power of AI while mitigating associated risks. The FDA’s framework provides a structured approach to navigate the complexities of AI in drug development, promoting safety, quality, and reliability.
As we move forward, it’s essential to recognize that balancing AI benefits and risks is an ongoing process. The FDA welcomes comments on their draft guidance until April 7, 2025, indicating the evolving nature of regulatory expectations in this field. This adaptive approach is crucial as we transition to examining the regulatory and governance considerations surrounding AI in drug development, which will be explored in the next section.
Regulatory and Governance Considerations
Now that we have explored the delicate balance between AI benefits and risks in drug development, let’s delve into the crucial regulatory and governance considerations that shape the landscape of AI implementation in this field.
A. Current legal frameworks and their limitations
The rapid advancement of AI in healthcare and pharmaceutical sectors has outpaced existing regulatory frameworks, creating a gap between innovation and governance. While some progress has been made, current legal structures often struggle to keep up with the pace of AI developments:
- European Union (EU): The AI Act, set to be implemented in August 2024, aims to establish a uniform legal framework for AI development and use. This act emphasizes:
- Safety and risk management
- Prohibition of high-risk AI practices
- Rigorous oversight and documentation for high-risk AI systems
- Transparency and security measures
- United States (US): Lacks comprehensive AI regulations but has taken steps through:
- FDA guidelines and action plans
- Focus on overseeing AI in medical devices and drug development
- Emphasis on public feedback and stakeholder engagement
Region | Key Regulatory Approach | Implementation Date |
---|---|---|
EU | AI Act | August 2024 |
US | FDA Guidelines | Ongoing |
Despite these efforts, limitations persist:
- Slow adaptation to rapid AI advancements
- Lack of global standardization
- Challenges in ensuring transparency in AI decision-making processes
- Difficulties in addressing ethical considerations comprehensively
B. Need for tailored AI algorithms in scientific research
The pharmaceutical industry recognizes the potential of AI to revolutionize regulatory affairs and scientific research. Tailored AI algorithms are crucial for:
- Simplifying and automating complex regulatory processes
- Improving efficiency in quality management
- Identifying vulnerabilities in drug development pipelines
- Enhancing data analysis and decision-making through machine learning (ML)
Key benefits of tailored AI algorithms include:
- Advanced data exploration capabilities
- Adaptability to various data types and ages
- Streamlined product development
- Insights into market dynamics
However, challenges remain:
- Robust validation of AI systems is essential
- Ensuring safety of AI-generated clinical endpoints
- Balancing AI integration with human expertise
C. Ensuring accountability in AI-generated content
As AI becomes more prevalent in drug development and healthcare, ensuring accountability for AI-generated content is paramount. Key considerations include:
- Transparency: AI decision-making processes must be transparent to allow for scrutiny and validation.
- Data integrity: The quality and integrity of input data significantly impact AI outcomes, necessitating rigorous data management practices.
- Ethical standards: Maintaining compliance with ethical standards in AI-generated content is crucial for patient safety and trust.
- Human oversight: Adequate clinician involvement and training for healthcare professionals are essential to mitigate risks of misuse or erroneous medical assessments.
Regulatory bodies are taking steps to address these concerns:
- The International Organization for Standardization (ISO) introduced ISO/IEC 42001:2023, providing a structured approach to managing AI risks and opportunities.
- The World Health Organization (WHO) has published regulatory considerations to guide safe AI usage in healthcare.
As we move forward, the focus shifts to ethical implementation strategies that can effectively address these regulatory and governance challenges. The next section will explore how stakeholders can navigate the complex landscape of AI in drug development while prioritizing patient safety, innovation, and ethical considerations.
Ethical Implementation Strategies
Now that we have covered the regulatory and governance considerations surrounding AI in drug development, let’s explore the ethical implementation strategies that can help mitigate risks and maximize benefits.
Promoting ethics-focused AI research initiatives
To address the ethical challenges in AI-driven drug development, it’s crucial to promote research initiatives that prioritize ethics. The GSK.ai-Stanford Ethics Fellowship is an excellent example of such an initiative, aiming to foster ethics-focused AI research in the pharmaceutical industry. These programs encourage researchers to:
- Develop AI algorithms tailored specifically for scientific writing and research
- Explore the ethical implications of AI decision-making in healthcare
- Address potential biases in AI-generated content
By supporting such initiatives, we can ensure that ethical considerations are at the forefront of AI advancements in drug discovery and development.
Integrating ethical considerations throughout the research process
Ethical implementation of AI in drug development requires a holistic approach that integrates ethical considerations from the outset of research projects. This approach involves:
- Ensuring informed consent and transparency in AI-driven clinical trials
- Maintaining patient data privacy and cybersecurity
- Addressing potential biases in AI algorithms and datasets
- Balancing the benefits of AI with potential risks to healthcare professionals and patients
Ethical Consideration | Implementation Strategy |
---|---|
Informed consent | Develop clear protocols for obtaining consent in AI-driven trials |
Data privacy | Implement robust data protection measures and encryption technologies |
Algorithm bias | Regularly audit AI models for potential biases and diverse representation |
Professional impact | Create programs to retrain and upskill healthcare professionals |
By integrating these ethical considerations throughout the research process, we can ensure that AI technologies in drug development are developed and implemented responsibly.
Anticipating both intended and unintended uses of AI technologies
As we integrate AI into drug development, it’s essential to anticipate and prepare for both intended and unintended consequences of these technologies. This proactive approach involves:
- Conducting thorough risk assessments of AI applications in drug discovery
- Developing contingency plans for potential misuse or unintended outcomes
- Establishing guidelines for responsible AI use in pharmaceutical research
Some key areas to focus on when anticipating AI technology uses include:
- Potential job displacement due to automation in drug discovery processes
- Unintended biases in AI-generated research outcomes
- Cybersecurity threats and data breaches in AI-driven clinical trials
- Ethical implications of AI decision-making in drug efficacy and toxicity predictions
By carefully considering these factors, we can better prepare for the ethical challenges that may arise as AI technologies become more prevalent in drug development.
As we move forward, it’s crucial to remember that ethical implementation strategies are not static but evolve alongside technological advancements. With this in mind, next, we’ll explore the impact of AI in drug development on healthcare professionals and patients, examining how these ethical considerations directly affect those at the forefront of medical care and treatment.
Impact on Healthcare Professionals and Patients
Now that we have explored ethical implementation strategies for AI in drug development, let’s examine the impact of these technologies on healthcare professionals and patients. This crucial aspect of AI integration in healthcare deserves careful consideration to ensure that the benefits of technological advancements do not come at the cost of human well-being and autonomy.
A. Potential job displacement due to automation
As AI technologies continue to advance in the healthcare sector, there is growing concern about potential job displacement for healthcare professionals. The integration of AI in various aspects of healthcare, from diagnostics to drug discovery, may lead to significant changes in the workforce landscape:
- Administrative tasks: AI can streamline processes, potentially reducing the need for administrative staff.
- Diagnostic imaging: Advanced AI algorithms may decrease the demand for radiologists and other imaging specialists.
- Drug discovery: AI-driven research could impact traditional roles in pharmaceutical research and development.
However, it’s important to note that while some jobs may be at risk, new opportunities are likely to emerge:
Job Category | Potential Impact | New Opportunities |
---|---|---|
Administrative | High risk of automation | Data analysis and AI system management |
Clinical | Moderate risk, focus on decision support | AI-assisted diagnostics and treatment planning |
Research | Shift towards AI-driven methodologies | AI model development and validation |
The World Health Organization (WHO) has highlighted the need to address these potential social gaps exacerbated by AI, which could lead to increased inequality, particularly in developing nations. It’s crucial to implement strategies that support workforce transition and upskilling to mitigate the negative impacts of job displacement.
B. Maintaining human elements of empathy and compassion
While AI offers significant benefits in healthcare, there is a growing concern about the potential loss of essential human interactions. The integration of AI in healthcare raises questions about maintaining empathy and compassion, which are vital for effective medical treatment:
- Patient-provider relationships: AI should complement, not replace, the human touch in healthcare.
- Emotional support: Certain medical fields, such as obstetrics and psychiatry, require high levels of emotional intelligence that AI currently cannot replicate.
- Holistic care: Human healthcare professionals can consider complex social and emotional factors that may be overlooked by AI systems.
To address these concerns, healthcare institutions should:
- Implement AI in ways that enhance, rather than diminish, human interactions
- Provide training for healthcare professionals on effectively integrating AI while maintaining empathetic care
- Regularly assess the impact of AI on patient satisfaction and overall care quality
C. Ensuring patient autonomy in AI-driven healthcare environments
As AI becomes more prevalent in healthcare decision-making, safeguarding patient autonomy becomes increasingly important. Key considerations include:
- Informed consent: Patients must be fully aware of how AI is being used in their care and have the right to make informed decisions.
- Data privacy: The General Data Protection Regulation (GDPR) in the EU and the Genetic Information Non-discrimination Act (GINA) in the US provide frameworks for protecting personal health data, but may need to be updated to address AI-specific concerns.
- Algorithmic transparency: Patients should have access to information about how AI systems make decisions that affect their care.
To ensure patient autonomy in AI-driven healthcare:
Develop clear guidelines for obtaining informed consent in AI-assisted medical procedures
- Implement robust data protection measures that go beyond current regulations
- Create patient education programs to help individuals understand and navigate AI-driven healthcare environments
The WHO emphasizes the importance of human dignity, transparency, inclusiveness, and sustainability in AI systems. By prioritizing these principles, healthcare providers can foster trust among patients and ensure that AI technologies serve as beneficial tools in enhancing global health outcomes while safeguarding privacy and ethical standards.

The ethical implications of AI in drug development present both exciting opportunities and significant challenges. As we’ve explored, AI has the potential to revolutionize the pharmaceutical industry by enhancing efficiency, accuracy, and speed in identifying novel compounds and predicting drug efficacy. However, these advancements come with crucial ethical considerations, including data privacy, informed consent, and the risk of exacerbating social inequalities.
Moving forward, it is imperative that stakeholders in healthcare, technology, and policy work together to establish robust regulatory frameworks and governance structures. These measures should prioritize patient safety, data protection, and equitable access to AI-driven healthcare innovations. By fostering collaboration between AI researchers and pharmaceutical scientists, and integrating ethical considerations throughout the drug development process, we can harness the transformative power of AI while safeguarding the human elements of empathy and compassion that are essential to medical care. As we navigate this rapidly evolving landscape, our ultimate goal must be to leverage AI responsibly, ensuring that its benefits in drug development truly serve the greater good of global public health.